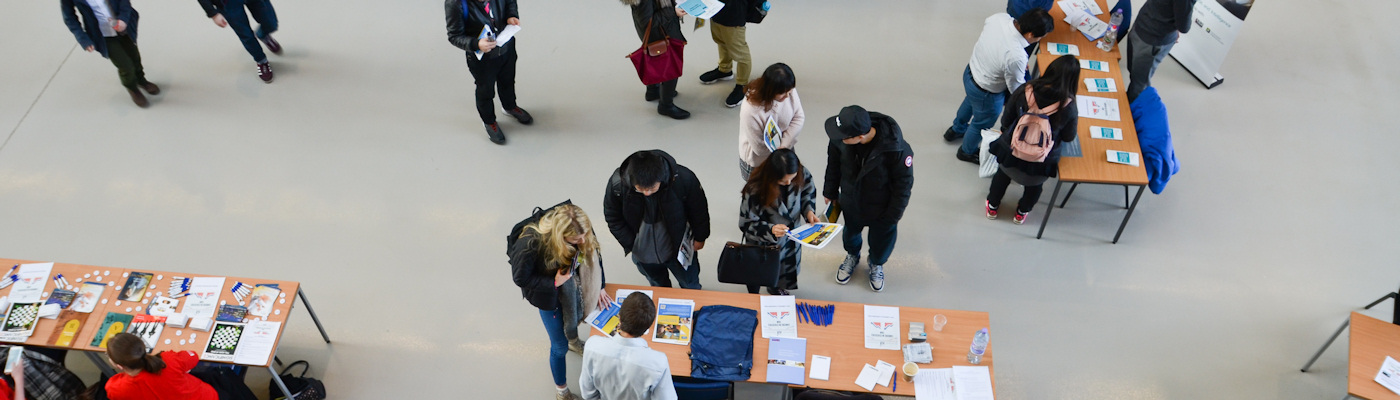
Events in the Department of Mathematics
Find out more about events, seminars and public lectures in the Department of Mathematics.
AI-Fun with ELLIS Seminar | Laurent Orseau
The Manchester Centre for AI Fundamentals and Manchester's ELLIS Unit are co-hosting a series of seminars featuring expert researchers working in the fundamentals of AI. Bio: Laurent has been working as a research scientist at DeepMind since 2014 having received a PhD in Artificial Intelligence from INSA Rennes / Université de Rennes II in 2007. Title...
Training Course on Visualisation & quantification of tomographic datasets
A 2 day in person training course covering theory and computer-based practical works using the Thermo Scientificâ„¢ Amira-Avizo Software. Lab/synchrotron X-ray tomography has emerged as one of the most important techniques for research in a wide range of applications including healthcare, energy, food and geology. Advances in the quality of X-ray...
Training Course on In situ experiments and Digital Volume Correlation
Digital Volume Correlation (DVC) is a powerful experimental technique that computes 3D full-field displacement and strain maps from volumes images acquired during a deformation process of a material. DVC is the 3D extension of Digital Image Correlation (DIC) which was first described four decades ago. The emergence of DVC started early 2000s with...
Training Course on Advanced Scripting and Batching of Tomographic Datasets
Although X-ray tomography is an attractive characterisation technique in materials science, it generates a huge amount of data at a fast rate and it can be extremely time consuming to process the data manually. A great benefit to creating scripts and plugins in Avizo is the ability to reuse a workflow on more than one image or to develop a bespoke...
Training Course on Visualisation & quantification of tomographic datasets
A 2 day in person training course covering theory and computer-based practical works using the Thermo Scientificâ„¢ Amira-Avizo Software. Lab/synchrotron X-ray tomography has emerged as one of the most important techniques for research in a wide range of applications including healthcare, energy, food and geology. Advances in the quality of X-ray...
- Page 1