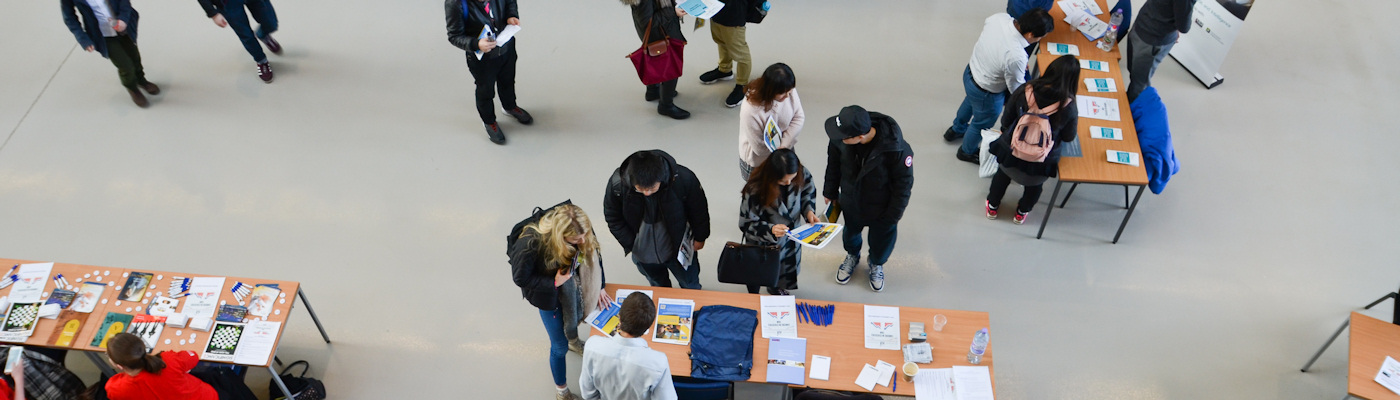
Events in the Department of Mathematics
Find out more about events, seminars and public lectures in the Department of Mathematics.
Chemical Imaging and Tomography - Training Course
Jumpstart your understanding of advanced chemical imaging & tomography with this three-day course by Finden who received the prestigious Royal Socity of Chemistry award in 2023 for pioneering the XRD-CT method. Learn about the fundamental principles, current instrumentation, and how these approaches are applied using the scattering and spectroscopic...
Dynamical Systems and Analysis Seminar - Diego Martinez
Speaker: Diego Martinez (University of Munster) Title: Generalized semigroup dynamics and their C*-algebras Abstract: Dynamical systems, that is, actions of (discrete) groups on locally compact spaces, have (classically) been used to construct broad classes of C*-algebras that are both interesting and manageable. In this talk we will discuss...
Manchester Geometry Seminar - Filippo Baroni
Speaker: Filippo Baroni (Oxford) Title: Algorithmic classification of surface homeomorphisms Abstract: Up to homotopy, homeomorphisms of closed surfaces come in three guises: periodic, reducible, and pseudo-Anosov. Among these three categories, pseudo-Anosov homeomorphisms exhibit qualitatively different topological, dynamical, and geometric...
Algebra seminar - Vlad Vankov
TBC
Digital Futures PhD Network
The Digital Futures network serves as a dynamic and collaborative hub exclusively designed for the community of PhD students within The University of Manchester, who are conducting research related to digital topics. The primary objective of this network is to help students to develop interdisciplinary, cross faculty relationships that can lead...
Manchester Number Theory Seminar - Josh Males
Speaker: Josh Males (University of Bristol) Title: TBA Abstract: TBA Room: Frank Adams 1
Giorgos Minas -- Efficient simulation, analysis and inference for large oscillatory stochastic systems [ONLINE]
Join us for this seminar by Giorgos Minas (St Andrews) as part of the North West Seminar Series in Mathematical Biology and Data Sciences The talk will be hosted by the University of Liverpool and available to watch via zoom. Please contact carl.whitfield@manchester.ac.uk or mdomijan@liverpool.ac.uk for the link, or sign up to the mailing list. Title:...
Logic seminar: Sylvy Anscombe
Title: Uniformities for Hilbert's Tenth Problem in henselian valued fields Abstract Hilbert's Tenth Problem in its original form asks for an algorithm to determine correctly whether -- or not -- a given multivariable polynomial equation with integer coefficients has integer solutions. The surprising resolution, by Davis, Putnam, Robinson, and...
Manchester Number Theory Seminar - Cassani
Speaker: Cassani Title: The Pila-Zannier strategy and the Zilber-Pink conjecture Abstract: I will explain how the Pila-Zannier strategy plays out in the context of the Zilber-Pink conjecture for connected pure Shimura varieties. This extends Daw-Ren2018 from algebraic curves to irreducible varieties of arbitrary dimension. Room: Frank Adams...
AI-Fun Seminar | Neill Campbell: Generative models as priors for inverse problems
The Manchester Centre for AI Fundamentals is hosting a series of seminars featuring expert researchers working in the fundamentals of AI. Title: Generative models as priors for inverse problems. Abstract: We will discuss the use of generative models in the regularisation of inverse problems involving both appearance and shape as well as the...